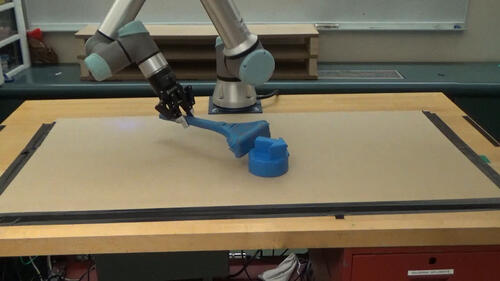
August 18, 2022
Lab member Meiying Qin defended her dissertation “Robot Tool Use: Learning, Transferring, Reasoning, and Applying Knowledge about Robots Using Human Tools“ today. Meiying will work as an assistant professor (teaching stream) at the Electrical Engineering and Computer Science department, Lassonde School of Engineering, York University. She will focus mainly on teaching, which includes giving lectures, developing pedagogy, designing new courses that emphasize experiential learning, and conducting research on Computer Science Education (CSE).
Abstract:
Using human tools can significantly benefit robots in many application domains. It will endow a home robot with the ability to carry out everyday household activities such as cleaning. It will enable an industrial robot to work in manufacturing or maintenance using human tools such as screwdrivers. It will allow a robot to perform experiments using existing human lab tools in a chemistry lab. Such ability would allow robots to solve problems that they were unable to without tools.
However, robot tool use is a challenging task. Tool use was initially considered to be the ability that distinguishes human beings from other animals (Oakley, 1944). Robot tool use has three challenges: perception, manipulation, and cognition. While both general manipulation tasks and tool use tasks require the same level of perception accuracy, there are unique manipulation and cognition challenges in robot tool use.
In this dissertation, we first define robot tool use and compile a taxonomy of robot tool use. We identify required skills for each sub-type in the taxonomy and review previous studies on robot tool use based on the taxonomy. Next, we demonstrate our work on solving some of the sub-types of robot tool use. We present an integrated system that trains a robot with tool use, transfers the learned skills to novel objects, and can even improvise the usage of novel tools. The robot that utilizes this system can also generalize the learned skills to other robot platforms without additional training. We then investigate more complicated forms of robot tool use. Specifically, we explore how a robot can plan sequential tool use so that the robot can use one tool to retrieve another tool in order to complete a task. We also examine how a robot should choose the most appropriate tool from many possible tool options. Finally, we investigate the application of learned tool use skills in related applications such as human-robot collaborations.
We conclude this dissertation with the contributions and limitations of our work. We also identify open challenges that remain to be solved as future directions.
Advisor: Brian Scassellati
Other committee members:
Aaron Dollar
Dragomir Radev
Goldie Nejat (University of Toronto)