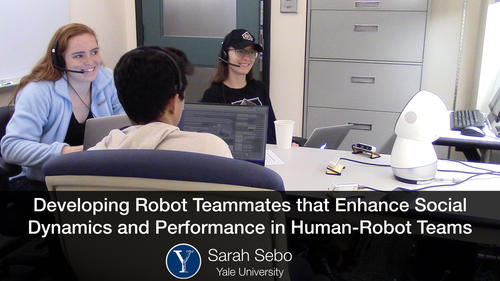
Lab member Sarah Sebo successfully defended her dissertation “Developing Robot Teamates that Enhance Social Dynamics and Performance in Human-Robot Teams.” Sarah will start as an assistant professor of Computer Science at University of Chicago in the fall.
Abstract:
Collaborative teams of people are most successful when they have positive social dynamics, where team members trust one another [Jones and George, 1998], feel included [Shoreet al., 2011], and feel comfortable to openly discuss mistakes and errors [Edmondson, 1999].Some of these social dynamics have even been shown to be more correlated with team performance than measures of individual intelligence [Woolley et al., 2010]. As robots increasingly join collaborative teams of people in a variety of settings (e.g., manufacturingplants, surgical suites, corporate workplaces, homes), it is essential that we build robotsthat can perceive and positively influence these social dynamics for the benefit of the team.
The work in this dissertation explores how social robots can enhance important socialteam dynamics in collaborative human-robot teams. It specifically investigates how robot behavior can positively shape trust, inclusion, and psychological safety, social dynamics that have been shown to have a significant positive influence on team performance. Our work demonstrates that a robot’s behavior can influence not just how people interact withthe robot, but how people in the group interact with each other. Finally, we investigate away in which robots may be able to sense social dynamics in real time, by perceiving human backchanneling behavior, and how social robots can shape this human social behavior in human-robot teams.
We investigate several robot behaviors (e.g., expressions of vulnerability, verbally sup-portive utterances) that have never previously been explored in the context of multi-person human-robot teams and demonstrate their ability to influence the team’s trust, inclusion,and psychological safety. We first explore the benefits of a robot asking pairs of childrentask-focused and relationship-focused questions to improve overall collaborative skill in a collaborative task, finding contrasting effects on task performance and perceptions of task performance. Next, we examine how to make the most effective trust repair in the context of a one-on-one human-robot interaction and highlight the benefits of a robot apologizingfor mistakes. Then we extend this idea to a group context and investigate how a robot’s expressions of vulnerability (including apologizing for and admitting to mistakes) might influence trust-related behavior in groups, showing that robot vulnerability increases trust-related behavior expression by people within the group towards one another as well as their conversational dynamics. Following this, we explore two strategies to improve humanteam member inclusion in a human-robot team and highlight the negative effects of giving someone in the group a specialized role to interact with the robot and the benefits ofrobot verbal support. Finally, we further analyze the role of robot verbal support withina human-robot team, demonstrating that robot verbal support may reduce the amount of verbal backchannel support human team members give one another.
We also suggest a method for perceiving group dynamics in real time through the recog-nition of human verbal backchannels from audio signals. Based on the data collected inone of our human-subjects experiments, we found significant positive correlations between verbal backchanneling behavior and participant ratings of psychological safety and inclusion. Thus, we believe that sensing human verbal backchannels can be a useful input for quickly perceiving social dynamics, enabling robots and other artificial agents to adapt their behavior based on real-time changes in social team dynamics.Taking into account all of our findings, we propose a set of guidelines for social robot behavior use in collaborative human-robot teams. In these guidelines, we recommend social robot behavior for particular situations and contexts, including tense interactions, robot errors and mistakes, teams where one or more members may have a specialized role, andinteractions where someone feels excluded. The combination of these guidelines, the resultsfrom our experimental studies, and the connections we made between backchannels andteam social dynamics make a significant contribution to building robots that can positively shape the social dynamics and performance of human-robot teams.